Predicting Academic Success of College Students Using Machine Learning Techniques
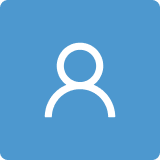
Round 1
Reviewer 1 Report
Comments and Suggestions for AuthorsThe article titled "Predicting academic success of college students using machine learning techniques" explores the use of machine learning (ML) techniques to predict academic success in college students. It investigates various preprocessing techniques, classification algorithms, and evaluates their effectiveness using a dataset containing academic and socio-economic information. The use of the CRISP-DM methodology ensures a systematic approach to data analysis, enhancing the study's reliability.
I suggest the following:
Introduction
Expand on the significance of academic success prediction within the broader context of educational improvement and policy reform. Highlighting the societal and economic impacts of educational attainment can provide a stronger motivation for the research.
More explicitly define the specific objectives and research questions at the outset to guide readers through the study's goals and expectations.
Methodology
Expand on the selection criteria for machine learning algorithms and the rationale behind the choice of preprocessing techniques. Including a comparison of alternative methodologies could strengthen the argument for the chosen approach.
Results
Discuss the statistical significance of the results to ensure the findings are not due to chance. Providing confidence intervals or p-values can enhance the credibility of the results.
Discusion
Elaborate on how the findings can be applied in educational settings. Discuss potential strategies for educational institutions to leverage these predictions for early interventions.
Suggest specific areas for future research, including the exploration of additional predictive factors, longitudinal studies to track changes over time, and the implementation of the findings in real-world educational interventions.
By continuing to explore and address the highlighted recommendations, the authors can not only enrich their current research but also pave the way for substantial future advancements in the field of educational data mining.
Best luck
Author Response
Dear Reviewer.
Thank you for giving us the opportunity to submit revisions. Your comments have led to valuable changes in the manuscript and the suggested revisions are attached.
Please see the attachment.
Kind regards,
The Authors.
Author Response File: Author Response.pdf
Reviewer 2 Report
Comments and Suggestions for AuthorsBased on the dataset examined consists of 6,690 records and 21 variables with academic and socio- 16 economic information,this work unveil the effectiveness of Educational Data Mining techniques, such as xgboost,RandomForest, and NaiveBayes; Moreover, the important variables underlying the predictive model of better performance are unveiled.
Major comments:
1. In the section of ‘3.3 Metric assessment’, some symbols in the formula need to be explained in detail, e.g. In Equation (4), what’s mean of ‘α’ and ‘β’.
2. Fig. 2 and Fig.4 need to be clearer.
3. More references highly related to this work are recommended, such as:
[1] Yuling M A , Cui C , Nie X ,et al.Pre-course student performance prediction with multi-instance multi-label learning[J]. SCIENCE CHINA Information Sciences, 2019, 62(2):3.
[2] Meier Y , Xu J , Atan O ,et al.Predicting Grades[J]. 2015.DOI:10.1109/TSP.2015.2496278.
Comments on the Quality of English LanguageThe writing is good.
Author Response
Dear Reviewer.
Thank you for giving us the opportunity to submit revisions. Your comments have led to valuable changes in the manuscript and the suggested revisions are attached.
Please see the attachment.
Kind regards,
The Authors.
Author Response File: Author Response.pdf