Deep Learning for Natural Language Processing (NLP) and Image Classification (IC)
A special issue of Applied Sciences (ISSN 2076-3417). This special issue belongs to the section "Computing and Artificial Intelligence".
Deadline for manuscript submissions: 30 June 2024 | Viewed by 580
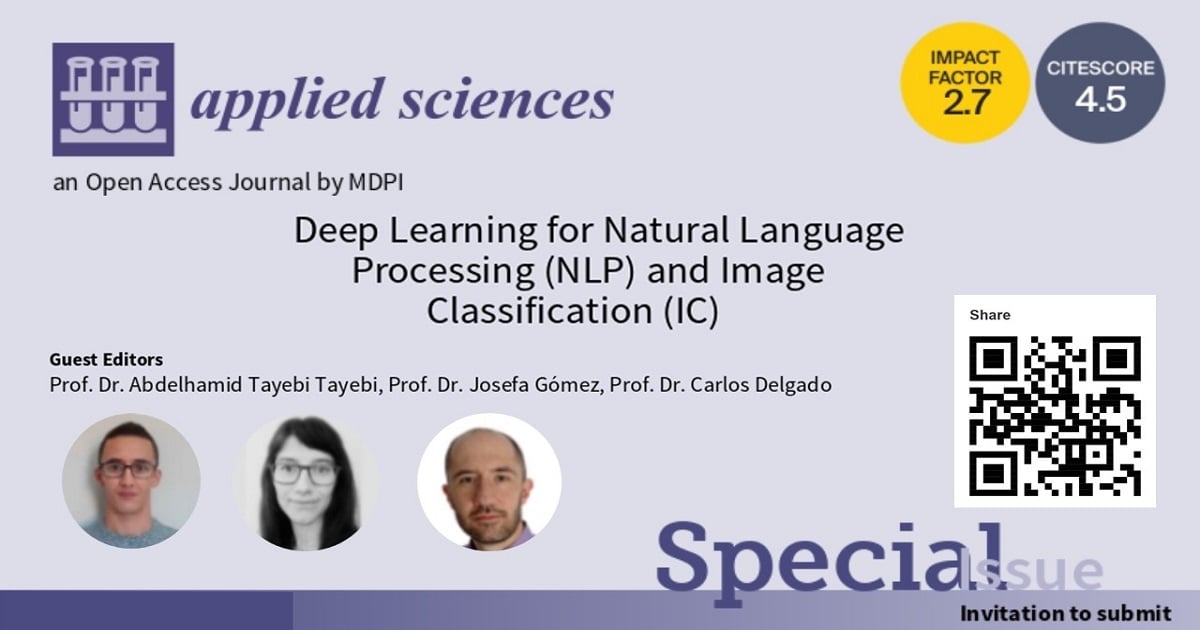
Special Issue Editors
Interests: artificial intelligence; deep learning; design and optimization of antennas; electromagnetic radiation and scattering
Special Issues, Collections and Topics in MDPI journals
Interests: artificial intelligence; deep learning; optimization and analysis of antennas; design of graphical user interfaces; propagation for mobile communications or wireless networks
Special Issue Information
Dear Colleagues,
This Special Issue will be an assemblage of the latest advancements in deep learning, particularly focusing on its applications in Natural Language Processing (NLP) and Image Classification (IC). The goal is to present a diverse range of novel ideal and empirical results, covering both theoretical foundations and practical implementations. This encompasses the utilization of artificial intelligence, machine learning, and deep learning for processing large datasets obtained from various sources such as satellites, scientific explorations, sensor networks, and medical diagnostics.
A part of this Special Issue is dedicated to the comparative of deep learning models. This involves a critical evaluation of these models in terms of their efficiency and accuracy across diverse datasets and real-world scenarios. The process aims to provide insights into the generalizability and interpretability of these models, thereby fostering advancements in their practical applicability.
Topics of interest include, but are not limited to, the following:
- Artificial intelligence tools and applications;
- Natural Language Processing;
- Speech recognition;
- Comparative of deep learning models;
- Intelligent system.
Prof. Dr. Abdelhamid Tayebi Tayebi
Prof. Dr. Josefa Gómez
Prof. Dr. Carlos Delgado
Guest Editors
Manuscript Submission Information
Manuscripts should be submitted online at www.mdpi.com by registering and logging in to this website. Once you are registered, click here to go to the submission form. Manuscripts can be submitted until the deadline. All submissions that pass pre-check are peer-reviewed. Accepted papers will be published continuously in the journal (as soon as accepted) and will be listed together on the special issue website. Research articles, review articles as well as short communications are invited. For planned papers, a title and short abstract (about 100 words) can be sent to the Editorial Office for announcement on this website.
Submitted manuscripts should not have been published previously, nor be under consideration for publication elsewhere (except conference proceedings papers). All manuscripts are thoroughly refereed through a single-blind peer-review process. A guide for authors and other relevant information for submission of manuscripts is available on the Instructions for Authors page. Applied Sciences is an international peer-reviewed open access semimonthly journal published by MDPI.
Please visit the Instructions for Authors page before submitting a manuscript. The Article Processing Charge (APC) for publication in this open access journal is 2400 CHF (Swiss Francs). Submitted papers should be well formatted and use good English. Authors may use MDPI's English editing service prior to publication or during author revisions.
Keywords
- machine learning
- deep learning
- artificial intelligence
- natural language processing
- speech recognition
- image classification
- comparative models
- algorithm efficiency
- real-world applications