Topic Menu
► Topic MenuTopic Editors
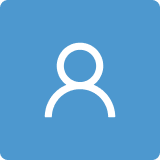
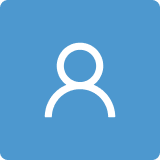
Big Data and Artificial Intelligence, 2nd Volume
Topic Information
Dear Colleagues,
The evolution of research in Big Data and artificial intelligence in recent years challenges almost all domains of human activity. The potential of artificial intelligence to act as a catalyst for all given business models, and the capacity of Big Data research to provide sophisticated data and services ecosystems at a global scale, provide a challenging context for scientific contributions and applied research. This Topic section promotes scientific dialogue for the added value of novel methodological approaches and research in the specified areas. Our interest is on the entire end-to-end spectrum of Big Data and artificial intelligence research, from social sciences to computer science including, strategic frameworks, models, and best practices, to sophisticated research related to radical innovation. The topics include, but are not limited to, the following indicative list:
- Enabling Technologies for Big Data and AI research:
- Data warehouses;
- Business intelligence;
- Machine learning;
- Neural networks;
- Natural language processing;
- Image processing;
- Bot technology;
- AI agents;
- Analytics and dashboards;
- Distributed computing;
- Edge computing.
- Methodologies, frameworks, and models for artificial intelligence and Big Data research:
- Towards sustainable development goals;
- As responses to social problems and challenges;
- For innovations in business, research, academia industry, and technology;
- For theoretical foundations and contributions to the bodyf knowledgef AI and Big Data research.
- Best practices and use cases;
- Outcomesf R&D projects;
- Advanced data science analytics;
- Industry-government collaboration;
- Systemsf information systems;
- Interoperability issues;
- Security and privacy issues;
- Ethicsn Big Data and AI;
- Social impactf AI;
- Open data.
Prof. Dr. Miltiadis D. Lytras
Prof. Dr. Andreea Claudia Serban
Topic Editors
Keywords
- artificial intelligence
- big data
- machine learning
- open data
- decision making
Participating Journals
Journal Name | Impact Factor | CiteScore | Launched Year | First Decision (median) | APC |
---|---|---|---|---|---|
![]()
Big Data and Cognitive Computing
|
3.7 | 4.9 | 2017 | 18.2 Days | CHF 1800 |
![]()
Economies
|
2.6 | 3.2 | 2013 | 21.4 Days | CHF 1800 |
![]()
Information
|
3.1 | 5.8 | 2010 | 18 Days | CHF 1600 |
![]()
Remote Sensing
|
5.0 | 7.9 | 2009 | 23 Days | CHF 2700 |
![]()
Sustainability
|
3.9 | 5.8 | 2009 | 18.8 Days | CHF 2400 |
MDPI Topics is cooperating with Preprints.org and has built a direct connection between MDPI journals and Preprints.org. Authors are encouraged to enjoy the benefits by posting a preprint at Preprints.org prior to publication:
- Immediately share your ideas ahead of publication and establish your research priority;
- Protect your idea from being stolen with this time-stamped preprint article;
- Enhance the exposure and impact of your research;
- Receive feedback from your peers in advance;
- Have it indexed in Web of Science (Preprint Citation Index), Google Scholar, Crossref, SHARE, PrePubMed, Scilit and Europe PMC.